Introduction to AI in Healthcare Diagnostics
Artificial Intelligence (AI) is transforming industries across the globe, but its role in healthcare—especially in diagnostics—is revolutionary. The healthcare sector has long relied on medical professionals to detect, analyze, and diagnose diseases based on patient data, physical examinations, and medical imaging. However, the complexities of diagnosing diseases leave room for human error, delays, and inefficiencies. AI is addressing these challenges by improving the accuracy, speed, and accessibility of diagnostics.
This article explores the role of AI in healthcare diagnostics, including its applications, advantages, challenges, and future possibilities. We’ll take a deep dive into how AI helps identify diseases early, provide faster and more precise diagnoses, and personalize treatments for patients around the world.
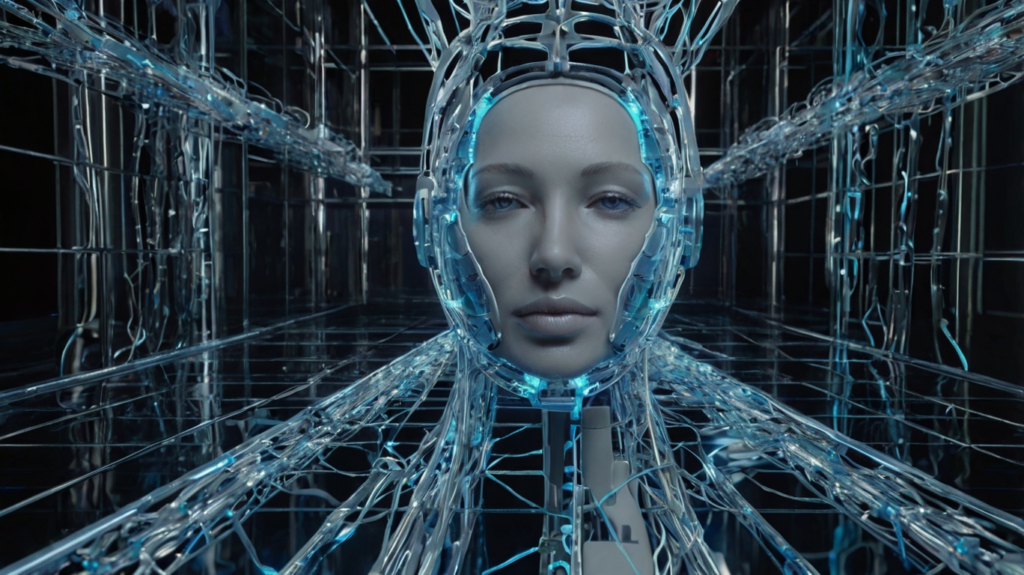
What is AI in Healthcare Diagnostics?
Artificial Intelligence refers to the simulation of human intelligence by machines. In healthcare diagnostics, AI algorithms analyze medical data such as X-rays, MRIs, CT scans, lab reports, and patient history to detect health issues. Unlike traditional methods, AI learns from large datasets and continues to improve its performance through machine learning (ML) and deep learning techniques. AI can identify patterns in data, predict health outcomes, and provide recommendations for treatment with remarkable precision.
AI systems work alongside doctors and healthcare professionals, providing decision support rather than replacing them. This collaboration ensures faster diagnoses while minimizing human error.
Importance of AI in Healthcare Diagnostics
1. Increased Accuracy in Diagnoses
AI algorithms outperform traditional diagnostic methods by identifying minute abnormalities in scans or test results. Human doctors, although highly skilled, are susceptible to fatigue or cognitive biases. AI tools can scan through large datasets efficiently, ensuring better detection of anomalies.
Example:
In radiology, AI can detect microcalcifications in mammograms—an early indicator of breast cancer—that may be too subtle for the human eye.
2. Faster Diagnostic Results
For life-threatening conditions, such as strokes or cardiac arrests, speed is critical. AI systems analyze test results or medical images almost instantly, delivering faster reports. This allows doctors to make timely decisions, especially during emergencies.
Example:
AI-based stroke detection systems can analyze brain scans in minutes, identifying blockages or bleeding in real-time, ensuring faster treatment.
3. Improved Accessibility to Quality Healthcare
In rural or remote areas where access to specialists may be limited, AI tools bridge the gap. Telemedicine platforms with AI diagnostic support enable local doctors to consult experts remotely or use AI tools to analyze patient data without delay.
4. Cost-Effective Solutions for Healthcare Providers
AI systems optimize workflows, reducing the need for unnecessary tests and minimizing hospital admissions. This efficiency translates to lower healthcare costs, benefiting both patients and healthcare institutions.
How AI Systems Work in Diagnostics
1. Machine Learning Algorithms in Diagnostics
Machine Learning (ML) involves training algorithms on large datasets to identify patterns and make predictions. In healthcare diagnostics, ML algorithms are trained on medical images, patient records, and laboratory results to predict conditions and recommend treatments.
- Supervised Learning: Trains the AI using labeled data, such as cancer vs. non-cancer images.
- Unsupervised Learning: AI identifies patterns on its own, revealing hidden trends or anomalies.
- Reinforcement Learning: AI improves itself through feedback, becoming more accurate over time.
Example:
ML-based software like Aidoc assists radiologists by flagging abnormal X-rays or CT scans that need further review.
2. Deep Learning for Image Analysis
Deep Learning, a subfield of ML, is particularly effective in analyzing complex data such as medical images. It uses neural networks—similar to how the human brain works—to process high-dimensional data and detect anomalies.
- Radiology: Detects fractures, tumors, and infections in X-rays and MRIs.
- Dermatology: Analyzes images of skin lesions to predict whether they are cancerous.
- Ophthalmology: Identifies signs of diabetic retinopathy through retinal images.
Applications of AI in Healthcare Diagnostics
1. AI in Radiology
Radiology involves interpreting medical images such as X-rays, MRIs, and CT scans. AI tools assist radiologists by scanning these images for abnormalities that indicate diseases like tumors, fractures, or infections.
Example:
Zebra Medical Vision has developed AI algorithms that analyze CT scans for signs of osteoporosis, aneurysms, and lung diseases. Their tools help radiologists make faster and more accurate diagnoses.
2. AI in Pathology
Pathology focuses on examining tissues and cells to diagnose diseases. AI tools analyze digital pathology slides, identifying cancer cells that may be missed by human pathologists.
Example:
Paige.AI developed an AI-powered tool that detects prostate cancer from biopsy slides with high accuracy. This tool reduces diagnostic errors and helps pathologists focus on critical cases.
3. AI in Cardiology
AI systems in cardiology analyze heart-related data, such as ECGs, to detect arrhythmias, heart attacks, and other cardiovascular issues. Predictive AI algorithms assess a patient’s risk of future heart events, allowing preventive measures.
Example:
The Mayo Clinic developed an AI tool that uses ECG data to predict asymptomatic heart disease, enabling doctors to take preventive steps even before symptoms appear.
4. AI in Ophthalmology
AI tools play a significant role in diagnosing eye diseases, including diabetic retinopathy, glaucoma, and age-related macular degeneration (AMD). By analyzing retinal images, AI provides early detection, preventing vision loss.
Example:
Google’s AI system for diabetic retinopathy achieved over 90% accuracy in detecting the disease, allowing for early treatment and preserving vision.
5. AI in Oncology
In oncology, AI is used to detect cancers early and recommend personalized treatment plans. AI-based systems analyze biopsy results, genetic profiles, and medical histories to recommend the best course of treatment.
Example:
IBM Watson for Oncology helps oncologists design treatment plans by analyzing clinical data and comparing it with the latest research and clinical trials.
Benefits of AI in Healthcare Diagnostics
- Accuracy and Consistency: AI tools deliver consistent results, reducing diagnostic errors caused by fatigue or cognitive bias.
- Faster Diagnoses: AI systems analyze data in real-time, reducing waiting times for patients.
- Proactive and Predictive Care: AI systems identify health risks early, allowing preventive interventions.
- Cost Savings: AI reduces the need for repetitive tests and unnecessary hospital visits.
- Personalized Treatment: AI algorithms create tailored treatment plans based on individual patient data.
Challenges and Limitations of AI in Diagnostics
1. Data Privacy and Security
AI systems require large datasets for training, raising concerns about patient privacy. Healthcare providers must ensure that sensitive data is protected from breaches and misuse.
2. Regulatory and Legal Issues
Regulating AI-powered diagnostic tools is challenging, as these systems need to meet high standards for accuracy and safety. Regulatory bodies like the FDA have to ensure AI tools are reliable before approval.
3. Algorithm Bias
AI systems can inherit biases present in the data they are trained on. If not addressed, these biases can lead to unequal healthcare outcomes for different patient groups.
4. Integration with Existing Systems
Implementing AI in healthcare facilities requires significant investment in infrastructure and training. Healthcare providers need to balance the cost of integration with the benefits AI offers.
5. Limited Human Interaction
Patients may feel uncomfortable when interacting with automated systems instead of human healthcare providers. AI must complement, not replace, human care to ensure patient satisfaction.
Future of AI in Healthcare Diagnostics
The future of AI in healthcare diagnostics looks promising. Here are some key trends to watch:
1. Wearable Devices for Continuous Monitoring
AI-powered wearable devices, such as smartwatches, monitor vital signs in real-time. They detect irregularities early and alert users to seek medical care.
2. Enhanced Telemedicine with AI Support
AI will play a crucial role in telemedicine by enabling remote diagnostics. Virtual consultations with AI-based diagnostic support will improve healthcare access in rural areas.
3. Genomic Analysis for Personalized Medicine
AI algorithms will analyze genetic data to identify mutations and predict genetic disorders. This will lead to more precise, personalized treatments for patients.
4. AI and Robotics in Surgery
Robotic surgeries assisted by AI will enhance precision and reduce recovery times for patients. AI will also support surgeons by providing real-time feedback during operations.
5. Predictive Analytics for Public Health
AI will help predict disease outbreaks and monitor population health trends. This data will enable governments and healthcare providers to plan preventive measures effectively.
Conclusion
AI is transforming healthcare diagnostics, making them faster, more accurate, and accessible. From radiology to oncology, AI tools are enhancing diagnostic capabilities across various medical fields. While challenges like data privacy and algorithm bias remain, continuous advancements will address these concerns. The collaboration between AI systems and healthcare professionals will result in better patient outcomes, reduced costs, and personalized care.
The future of healthcare diagnostics is bright, with AI playing a pivotal role in preventive care, personalized medicine, and global healthcare accessibility. As this technology evolves, it will not only improve diagnostic accuracy but also ensure that quality healthcare becomes a reality for all.
Key Takeaways
- AI enhances diagnostic accuracy and speed, reducing errors and saving lives.
- Applications of AI span radiology, cardiology, ophthalmology, oncology, and pathology.
- Early detection, cost savings, and personalized care are significant benefits of AI-powered diagnostics.
- Challenges include data privacy, regulatory hurdles, and algorithm bias.
- AI’s future lies in wearable technology, telemedicine, and predictive healthcare solutions.
With the right balance of technology and human care, AI will revolutionize diagnostics, shaping the future of medicine and improving lives worldwide.